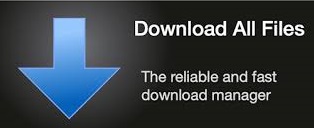
- #ADOBE MEDIA ENCODER CC 2015 CUDA PERFORMANCE MANUAL#
- #ADOBE MEDIA ENCODER CC 2015 CUDA PERFORMANCE VERIFICATION#
Although these methods can obtain in situ non-destructive images of roots by adjusting and combining different imaging parameters, they cannot enable the observation of larger plants, owing to expense and technical difficulty. To address this limitation, non-destructive observation methods such as X-ray computed tomography (CT) ( Mairhofer et al., 2012, 2013 Mooney et al., 2012), nuclear magnetic resonance (NMR) imaging technology ( Pflugfelder et al., 2017), laser scanning ( Fang et al., 2009), and 3D imaging ( Iyer-Pascuzzi et al., 2010 Clark et al., 2011 Topp et al., 2013) have been applied. Accordingly, these destructive sampling methods do not enable phenotypic observations of dynamic root systems in situ. Traditional root phenotype research methods mostly use the root drilling and soil column methods as well as other excavation methods ( Joslin and Wolfe, 1999 Wasson et al., 2016), followed by washing, screening, dyeing, scanning, and other necessary steps. However, soil is a non-transparent medium, so it is difficult to quickly and accurately obtain phenotypic information, which is a critical obstacle to research on root development. The growth environment of plant roots within the soil is extremely complex.
#ADOBE MEDIA ENCODER CC 2015 CUDA PERFORMANCE MANUAL#
Thus, using the improved DeepLabv3+ to segment root systems based on micro-root images is an effective method for accurately and quickly segmenting root systems in a homogeneous soil environment and has clear advantages over traditional manual segmentation. Based on the comparison of our segmentation results with those of traditional manual and U-net segmentation methods, this novel method can more accurately segment root systems in complex soil environments. The Spearman rank correlation between the manually obtained Rhizotrons manual segmentation root length and automated root length was 0.9667 ( p < 10 –8), with r 2 = 0.9449.
#ADOBE MEDIA ENCODER CC 2015 CUDA PERFORMANCE VERIFICATION#
After 80 epochs of training, the final verification set F1-score, recall, and precision were 0.9773, 0.9847, and 0.9702, respectively. Segmentation performance of the proposed method utilizing WinRHIZO Tron MF analysis was assessed using these images. We have improved the upsampling portion of the DeepLabv3+ network and validated it using in situ images of cotton roots obtained with a micro root window root system monitoring system. Here, we investigate the effectiveness of an automated image segmentation method based on the DeepLabv3+ convolutional neural network (CNN) architecture to streamline such measurements. This type of image segmentation method is extremely inefficient and very prone to human error. Root systems can be segmented from root images obtained by the Rhizotrons method, and then, root system lengths and diameters can be obtained with software. At present, researchers mostly use direct manual drawings or software-assisted manual drawings to segment root systems for analysis. However, the segmentation of root images is a critical obstacle restricting further development of this method. The Rhizotrons method is an important means of detecting dynamic growth and development phenotypes of plant roots.
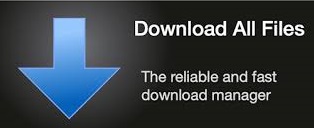